

His research is focused on decisionmaking in the face of uncertainty, including bandit algorithms and reinforcement learning.
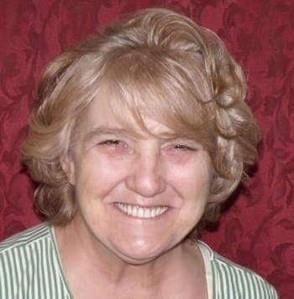
tor lattimore is a research scientist at DeepMind. The book ends with a peek into the world beyond bandits with an introduction to partial monitoring and learning in Markov decision processes. Linear bandits receive special attention as one of the most useful models in applications, while other chapters are dedicated to combinatorial bandits, ranking, non-stationary problems, Thompson sampling and pure exploration.
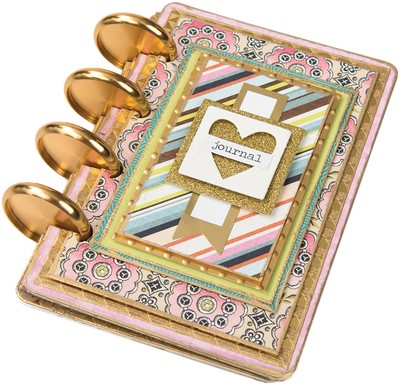

A focus on both mathematical intuition and carefully worked proofs makes this an excellent reference for established researchers and a helpful resource for graduate students in computer science, engineering, statistics, applied mathematics and economics. This comprehensive and rigorous introduction to the multi-armed bandit problem examines all the major settings, including stochastic, adversarial and Bayesian frameworks. Part IV Lower Bounds for Bandits with Finitely Many Armsġ3.1 MainIdeas Underlying Minimax Lower Boundsġ8.1 Contextual Bandits: One Bandit per ContextĢ0 Confidence Bounds for Least Squares EstimatorsĢ0.1 Martingales and the Method of MixturesĢ1 Optimal Design for Least Squares EstimatorsĢ2 Stochastic Linear Bandits with Finitely Many ArmsĢ3 Stochastic Linear Bandits with SparsityĢ4 Minimax Lower Bounds for Stochastic Linear BanditsĢ5 Asymptotic Lower Bounds for Stochastic Linear BanditsĢ5.1 AnAsymptotic Lower Bound forFixed Action SetsĢ7.1 Exponential Weights for Linear BanditsĢ8 Follow-the-regularised-Leader and Mirror DescentĢ9 The Relation between Adversarial and Stochastic Linear BanditsĢ9.2 Reducing Stochastic Linear Bandits toAdversarial Linear BanditsĢ9.3 Stochastic Linear Bandits withParameter Noiseģ0.4 Semi-bandit Feedback and MirrorDescentģ3.2 Best-Arm Identification witha Fixed Confidenceģ3.3 Best-Arm Identification witha Budgetģ4.1 Statistical Decision Theory and Bayesian Learningģ4.2 Bayesian Learning and thePosterior Distributionģ4.3 Conjugate Pairs, Conjugate Priorsand theExponential Familyģ5.1 Bayesian Optimal Regret for k-ArmedStochastic Banditsģ7.1 FiniteAdversarial Partial Monitoring Problemsģ7.3 Classificationof FiniteAdversarial Partial Monitoringģ8.2 Optimal Policies and theBellman OptimalityEquationģ8.4 Learning inMarkov Decision Processesģ8.5 Upper Confidence Bounds for Reinforcement Learningīandit Algorithms Decision-making in the face of uncertainty is a significant challenge in machine learning, and the multi-armed bandit model is a commonly used framework to address it. Part III Adversarial Bandits with Finitely Many Arms Part II Stochastic Bandits with Finitely Many ArmsĨ The Upper Confidence Bound Algorithm: Asymptotic Optimalityĩ The Upper Confidence Bound Algorithm: Minimax Optimalityġ0 The Upper Confidence Bound Algorithm: Bernoulli Noiseġ0.1 Concentration forSums of Bernoulli Random Variables Part I Bandits, Probability and ConcentrationĢ.1 Probability Spaces and Random ElementsĤ.7 The Canonical Bandit Model forUncountable Action Setsĥ.2 The Inequalities of Markov and Chebyshevĥ.3 The Cramér-Chernoff Method and Subgaussian Random Variables
